In such an emergence, a study conducted by PMC gives us an insight into the use of Artificial Intelligence accomplishing the task in question. The goal here was to formulate and analyze an automated structure that actively detects COVID-19 with the help of chest CT.
Introduction to the Study
The novel Coronavirus, with its extreme contagiousness, has spread across the world like wildfire on steroids. Its direct target is the human respiratory system, resulting in acute respiratory issues or even organ failure. It was declared as a “public health emergency of international concern” on January 30, 2020, by the World Health Organization (WHO).
The mechanism of determining that a patient is positive for COVID-19 or not mostly revolves around the reverse-transcription polymerase chain reaction (RT-PCR). But, sensitivity concerns with the chain reaction has led to inefficiency in terms of detection in the early stages, and the treatment that follows accordingly.
On the other hand, we have Computed Tomography (CT) that functions as an imaging system. This is why CT could prove to be more proficient in the diagnosis of COVID-19 as its capable of catching any irregularities accumulating in the lungs.
Nevertheless, as there are many diseases out there that attack the human lungs, CT could show similarities in imaging between different conditions and issues, making it hard to pinpoint COVID-19.
This is why the focus goes towards the quick-approaching Artificial Intelligence, which has made its way quite profoundly in the vast domain of medical treatment technology. The credit goes towards the use of deep learning technology, which is quite triumphant in feature extraction- a process of reducing data to the point where it’s only sufficient and valuable in machine learning and image processing.
Therefore, this study presents a 3D deep learning structure that actively detects and diagnoses COVID-19 using the helpful chest CT. This proposition is termed as COVID-19 detection neural network (COVNet). To test the authenticity here, Community-Acquired Pneumonia (CAP), along with several other non-pneumonia tests were included in the study.
The Methodology Used
After approval by the ethics committee of the hospitals involved, the study involved 4,536 3D volumetric chest CT exams from 3,506 patients gotten from six different medical institutes.
Upon applying the exclusion criteria, 4,356 3D chest CT exams were finalized. The age of the patients ranged from 34-49, and the study had faintly more male patients than female ones.
In the end, we have a total of 1296 (30%) exams for COVID-19, 1735 (40%) for Community-Acquired Pneumonia, and 1325 (30%) for non-pneumonia. Furthermore, all the COVID-19 patients were confirmed of their positivity through the polymerase chain reaction.
All the patients for this cause were chosen arbitrarily.
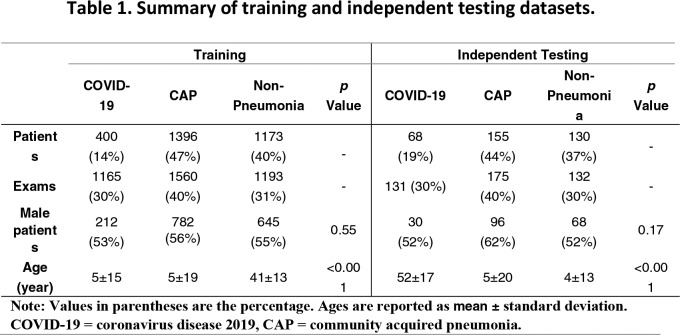
Patient Statistics
The CT tests were done by using numerous manufacturers, but with the basic imaging protocol. Moreover, the deep learning framework coined as COVNet was developed and can impart both 2D and 3D features from the exam.
Besides, the building block of the deep learning system is RestNet50, which analyzes multiple CT slices, and makes features out of them. These corresponding features are then indulged in a pooling operation that leads to a softmax activation function. This, in turn, produces an estimated score for each type of disease- COVID-19, CAP, and non-pneumonia.
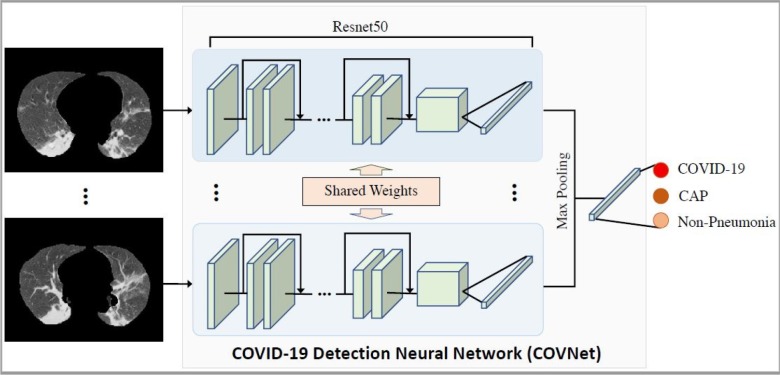
Deep Neural Tech
Project Results
Based on De Long’s method, the deep learning framework’s performance was assessed, and by using an independent testing method. A receiver operating characteristic (ROC) graph was plotted, along with the calculation of area under graph (AUC) by using confidence intervals (CIs).
It was then evaluated that the deep learning technology developed performs exceptionally well in terms of speed. The estimated average time for processing speed is 4.51 seconds with the proper equipment. In this case, GPU NVIDIA Quadro M4000 8GB, with RAM 16GB and Intel Xeon Processor E5-1620 v4 running at 3.5GHz, was used.
The performance summary can be seen in the table below. For COVID-19, the sensitivity is measured at about 90% combined with 95% CIs. The rest of the statistics can be seen in the table.
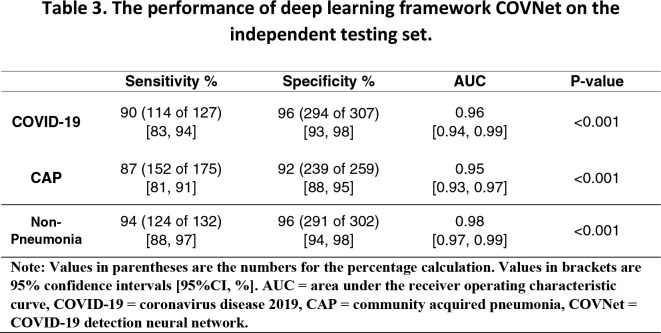
Stats For the Deep Learning Framework
Additionally, it was found out that patients confirmed with COVID-19 and CAP are older as compared to the ones in the non-pneumonia category ( COVID-19: 53; CAP: 51; Non-Pneumonia: 41).
However, not everything was picture-perfect. In extremely complicated cases, there are possible misinterpretations. Two of the figures below highlight how COVID-19 has been mistaken for CAP, and quite similarly, CAP has been mistaken for Coronavirus.
To better the precision here, a helpful suggestion is to include the history of the exposure. This narrows down the possibilities.
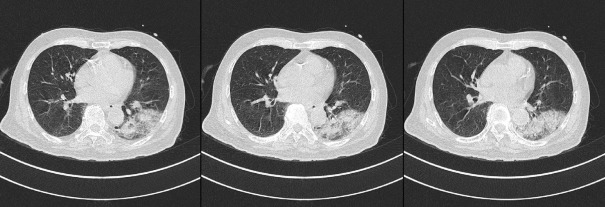
Pneumonia Misinterpretation
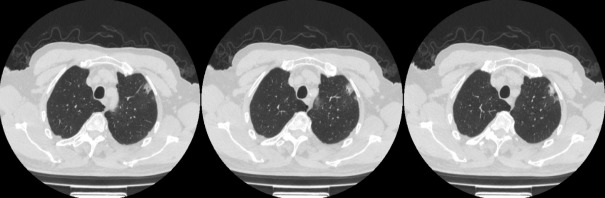
COVID Misinterpreted
Summary
As diagnosing Coronavirus has become an increasing problem over time, the study at hand focuses on aiding the world in creating an easy solution for detection.
Previous uses of Artificial Intelligence in the world of medicine significantly bore fruit. The techniques of the deep learning system have been thoroughly used to detect pneumonia in pediatric chest radiographs. That’s not it, they’ve also differentiated viral and bacterial pneumonia in 2D pediatric chest radiographs.
Keeping in mind the convenience and resolving power, this comprehensive study took a good amount of CT exams from various hospitals. These were 296 COVID-19 CT, 1735 CAP, and 1325 non-pneumonia exams. The tests taken other than COVID-19 were intentionally done so to measure the precision of the deep learning framework.
Work-a-ahead
Nevertheless, there are quite a few limitations to the study. First of all, the novel Coronavirus causes symptoms that may appear to be similar to other virus-caused diseases such as pneumonia concerning imaging. Due to a lack of etiology here, Community-Acquired Pneumonia was randomly selected, and that too from August 2016 to February 2020.
Secondly, there is a certain degree of transparency present in the technique of deep learning. A heat map was surely used, but it was not enough to distinguish contrasting features with precision. This is a drawback to using the deep learning mode of operation.
Furthering the limitations, the human lung considerably reacts to a high number of factors that may be rooting from age, underlying conditions, or drugs. A single method won’t be able to differentiate between lung peculiarities based on CT imaging.
Thus, the study promises to strive more in this regard; take even more CT exams from different health centers to accurately evaluate the model’s performance, and determine a reliable solution for the detection of COVID-19. They also shed light on the fact that their continued research will also showcase the severity degree of the Coronavirus, and not just its detection.
Conclusion
To conclude it all, Artificial Intelligence has indeed proved useful in terms of COVID-19 detection. It has, to a reasonable extent, distinguished COVID-19 from CAP in chest CT scans. This only shows how the approach of machine learning can undergo progress to yield even better results shortly.